Özyeğin Üniversitesi, Çekmeköy Kampüsü Nişantepe Mahallesi Orman Sokak 34794 Çekmeköy İstanbul
Telefon : +90 (216) 564 90 00
Fax : +90 (216) 564 99 99
info@ozyegin.edu.tr
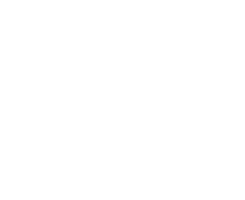
Thesis Defense - Sezin Ekşioğlu (MSDS)
Sezin Ekşioğlu – M.Sc. Data Science
Assoc. Prof. Okan Örsan Özener – Advisor
Date: 25.05.2022
Time: 12:30-13:30
Location: AB1 232
MULTILABEL CLASSIFICATION WITH NEURAL NETWORK
Thesis Committee:
Assoc. Prof. Okan Örsan Özener, Özyeğin University
Assoc. Prof. Uğur Çelikyurt, Koç University
Asst. Prof. Başak Altan Özener, Özyeğin University
Abstract:
Multi-label classification has huge importance for several applications, it is also a challenging research topic. It is a kind of supervised learning that contains binary targets. The distance between multilabel and binary classification is having more than one class in multilabel classification problems. Features can belong to one class or many classes. There exists a wide range of applications for multi-label prediction such as image labeling, text categorization, gene functionality. Even though features are classified in many classes, they may not always be properly classified. There are many ensemble methods for classification. However, most of the researchers have been concerned about better multi-label methods. Especially little ones focus on both efficiency of classifiers and pairwise relationships at the same time to implement better multi-label classification. In this paper, we worked on modified ensemble methods by getting benefits from k-Nearest Neighbors and neural network structure sequentially to address issues beneficially and to get better impacts from the multi-label classification. Publicly available datasets (yeast, emotion, scene, and birds) are performed to demonstrate the developed algorithm efficiency, and the technique is measured. Our algorithm outperforms benchmarks for each dataset with different metrics. The result of the algorithm is competitive with the state-of-the-art results. Especially, in the weighted average of false-positive minimization and false-negative minimization, the algorithm passes the benchmarks.
Bio:
Sezin Ekşioğlu graduated from Özyeğin University with a Computer Science degree in 2019. After graduation, she worked for Vodafone Turkey as a backend developer and Getir as a data scientist. Her main area of research interest is ensemble classification strategies. Besides she is currently involved in projects which are related to stacked classification methodologies based on data engineering. She also has experience in customer correlation studies for e-commerce fields.